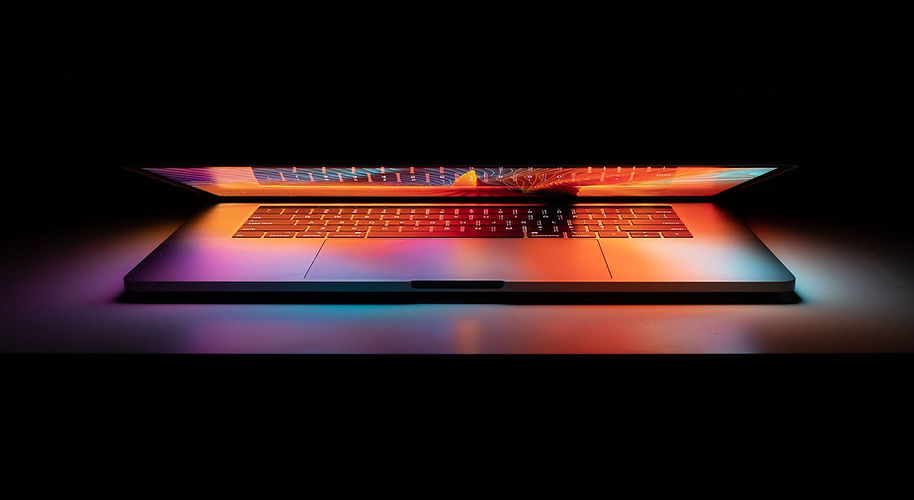
ETHICAL AI FRAMEWORK:
PRINCIPLES & POLICY
Effective starting: 20 August, 2022
​
Preamble
Artificial Intelligence1 (AI) has the potential to greatly enrich the human condition. The very fact of this massive potential means it also comes with the risk of harm, intended or not.
​
Plaetos Inc (Plaetos) uses AI systems extensively to deliver human insight. It is critical to Plaetos that we develop and implement AI systems in our technologies that are safe, secure and reliable.
​
Plaetos understands and acknowledges the potential risks associated with our use of AI systems, and the need for confidence by our customers and regulators that there is a management regime in place to ensure those systems are used responsibly. The benefits and enrichment to human endeavor that using AI brings cannot be achieved without active management of these potential risks.
​
This document presents Plaetos’ approach to deploying AI responsibly.
Core application of AI in PlaetosEQ
The foundational use of AI in the Plaetos applications is to reduce the human brain’s cognitive overload of processing masses of word data.
​
Use of AI in the Plaetos applications is currently restricted to natural language processing (NLP) to find useful signals within employee-generated text content. These signals are used to: (i) present the most relevant content in response to user queries through search, (ii) to present visualizations, and (iii) support content filtering and sorting, ie. AI with a “human-in-the-loop” approach.
Our Approach to Ethical AI
Our approach is to:
-
Be accountable for how we use AI by committing to and operating in accordance with this Ethical AI Principles and Policy document
-
Influence the development of ethical AI frameworks through contributing to and adopting industry and societal standards where applicable, and
-
Ensure relevant stakeholders understand how we deploy AI to inform their own risk assessments.
​
Plaetos Ethical AI Principles
Our use of AI is guided by the following principles:
​
1. TRANSPARENCY
Plaetos will be clear and consistent in informing users when AI is employed in our technologies; the intent of the AI; the model class; the data demographics; and the security, privacy, and human rights controls applied to the model in a manner that is accessible, transparent, and understandable. We will also share how to get more information about our use of AI.
2. NO HARM
Plaetos strives to identify and remediate any harmful bias within our algorithms, training data, and applications that are directly involved in consequential decisions; that is, decisions that could have a human rights, reputational or legal impact on individuals, groups or customer organizations.
3. ACCOUNTABILITY
Plaetos is committed to upholding and respecting the human rights of all people. The Plaetos Responsible AI Framework requires teams to account for privacy, security, and human rights impacts from the very beginning of development through the end of the AI lifecycle.
4. PRIVACY
Plaetos has built privacy engineering practices in the PlaetosEQ platform. These practices help ensure that we design, build, and operate privacy-protecting features, functionality, and processes into our product.
When processing personal information, Plaetos is committed to following the principles set forth in our Privacy Policy, which aligns with applicable international privacy laws and standards. Plaetos technologies are designed to operate without revealing Personal Identifiable Information (PII).
5. RELIABILITY
Plaetos designs and tests AI systems and their components for reliability. As part of our responsible AI assessment and monitoring, we design our model life-cycles for continuous improvement and monitor AI-based solutions for consistency of purpose and intent when operating in varying conditions.
6. RISK BASED
Plaetos takes a risk-based approach to the AI systems it designs. Risks are identified, mitigated and reviewed under a continuous accountability framework in accordance with our Risk Assessment Policy. We maintain a risk register that includes risks associated with AI systems and their components.
External Guidance and Commitments
Plaetos recognises that it operates within a dynamic social and business environment that is still coming to terms with the potential benefits and risks of AI. We seek guidance from AI ethics experts as they work towards a universal framework and certification regime for global AI risk management and accountability.
The organizations we look to for both internal standards setting and societal expectations include:
-
The World Economic Forum’s Principles and Operationalizing Principles for Responsible AI
-
UNESCO Recommendation on the Ethics of Artificial Intelligence
Summary of Plaetos’ AI and Machine Learning functions
Use of AI in the Plaetos applications is currently restricted to natural language processing (NLP) to find useful signals within employee-generated text content. These signals are used to present the most relevant content in response to user queries through search, to present visualizations and support content filtering and sorting, ie. AI with a “human-in-the-loop” approach.
​
Plaetos algorithms are deployed through a Machine Learning Pipeline, which performs a series of analyses when new text is imported to a customer’s PlaetosEQ instance. The ML pipeline and some models can be tailored for specific customers and information domains.
​
Specifically, our AI/ML applications are:
Text segmentation
Imported documents are segmented into semantically discrete sub-documents (chunks) by a segmentation algorithm. This improves the quality of NLP analysis and reduces problems associated with “averaging out” of sentiment and the generation of numerous, diverse topics from long documents. The relationship between chunks is maintained in the PlaetosEQ Graph.
Semantic search
Semantic search (ie. search based on meaning) is combined with faceted search (search constrained by document metadata or NLP analysis). Search is combined with semantic re-ranking to bring the most relevant content to the top of the search results page.
Topic modeling (pre-trained)
Pre-trained topic models are used to assign topics to documents to support data visualization and exploration. A top-level topic model provides the high-level topics (eg. work, finance, health). Finer-grained topics are provided through sub-topic models (eg. work subtopics: compensation, training & development, people to work with…) in the domains most relevant to our customers.
​
Topic modeling (ML)
Topic modeling using a machine learning clustering algorithm is used in some cases (eg. Plaetos Strategy Maps) to identify semantically similar content to support visualization and exploration.
Entity recognition
A pre-trained model is used to identify named entities (people, places, things). This analysis is combined with sentiment for visualization purposes, which enables users to see what’s being discussed positively/negatively.
Sentiment analysis
Sentiment models ascribe polarity to content based on language. Plaetos uses both 5-point and 3-point sentiment models. Sentiment is used for visualizing and filtering content. It is combined with entities to create “sentimented entity” visualizations and topics for “sentimented topic” visualizations.
Emotion analysis
A 16-point emotion model mapped to categories from Plutchik’s Wheel of Emotion provides a more nuanced assessment (beyond sentiment) of employee emotion expressed in their content. The outputs of this algorithm are combined with other analyses to support visualization and exploration.
Problems & Solutions
A problem/solution model categorizes content as containing statements about “good things”, “bad things” and “solutions”. It can be combined with other models to identify content such as “problems relating to compensation”.
Powerful Statements
A powerful statements model scores text documents according to their level of personal narrative power. This model is used to surface rich content suitable for quoting and illustrating particular points.
​
Summarization
We use both extractive and abstractive summarization to provide customers with concise summaries of content of interest.
Training Protocol for Machine Learning Algorithms
As most models used within Plaetos are deep learning models, they generally need to be pre-trained on relevant text content. Depending on the model being designed, training can be undertaken from scratch using a large training document set. Alternatively, the prior learning of a large language model (eg. BERT, GPT-3) can be adapted to be more accurate for our specific purposes through training on a smaller, more relevant, dataset using transfer learning.
​
Irrespective of the model being developed and the training process, the Training Protocol is the same and is guided by the Plaetos Ethical AI Policy and Principles. The Training Protocol recognises that all data contains biases but some biases are more important than others depending on how the model will be used.
​
Wherever possible, Plaetos sources and curates its own training datasets so that biases are well understood and can be mitigated where necessary.
​
Model Training Protocol:
-
The Model Scope document shall include details of model training process and training data, including the information covered in points 2-4 below.
-
Know and be able to describe the source(s) and composition of the training data
-
Know and be able to describe the main biases inherent in the training data (eg. gender, culture, age group, political affiliation…)
-
Select training and test datasets that are most appropriate for the model being developed and where known biases will have least impact on the quality of outputs
-
Document all model training runs (source data, quality of output) and retain this information in a secure, accessible form for subsequent review and audit
-
Results of model test runs must be reviewed by at least one suitably experienced person (the Reviewer) not closely involved with the model development. Multiple reviews shall be undertaken until the Reviewer is satisfied with the quality and consistency of the model outputs.
-
All models shall be signed off by the Plaetos CTO or their delegate before being deployed to a production environment.
​
​
Privacy Protections within PlaetosEQ
The PlaetosEQ platform is designed to operate optimally without exposing personal identifiable information (PII) or allowing an individual to be associated with any specific content or analysis.
​
The privacy protections within PlaetosEQ are multi-tiered and incorporate policy, contract and technical layers, which are explained below.
​
1. Plaetos Privacy and Information Security Policies
Plaetos maintains Privacy policies in conformance with the General Data Protection Regulation and Information Security policies in conformance with the SOC2 information security standard.
​
2. Plaetos Customer Contracts
Plaetos’ customer contracts state explicitly that Plaetos technologies: (i) are not designed to provide insights at the level of individual employees, (ii) contain active protections to ensure employee privacy is protected and (iii) that Plaetos will not attempt to re-identify employees except where required to do so by law.
​
3. Treatment of PII in Customer Content (technical)
The first step in the Plaetos Machine Learning Pipeline is identification and removal of Personal Identifiable Information (PII) and its replacement with a <PII> flag. The Plaetos PII removal process is built on top of the Microsoft Presidio data protection and anonymization library and is constantly updated to improve its accuracy without compromising data quality through excessive false alerts. PII removed includes: name, email, telephone number, social address, credit card number, SSN and TFN. This PII removal process is under constant review to improve its performance.
​4. Pseudo-anonymization (technical)
Employee identities (eg. email, employee number, application user ID) are needed in the ingested data so that demographic and organizational metadata can be correctly linked to employee content. These identities are pseudo-anonymized using a 1-way hash function. This allows future content to be linked to the same employee ID but does not allow reverse-engineering of the ID to identify the employee. Protections from re-identification (see below) operate in addition to pseudo-anonymization to achieve effective anonymization.
5. ​Protection from Re-identification (technical)
Technical protections within PlaetosEQ are being progressively implemented to prevent employees being re-identified based on demographic or organizational data associated with their content. Protections implemented include: employee ID (original & hashed) cannot be viewed through the user interface (UI); all linked demographic & organizational data is obscured when the number of employee IDs in a document set falls below N (with N set at 5 as a default minimum value that can be increased based on data sensitivity and customer requirements).
-------
​
1. In this Policy document, Artificial Intelligence is used to describe computer systems able to perform tasks normally requiring human intelligence such as reading and writing text. It incorporates Machine Learning and Deep Learning.